In the ever-evolving landscape of artificial intelligence, two paradigms stand out for their transformative impact on industries and society: Generative AI and Traditional Machine Learning. While both harness the power of data to drive innovation, they differ fundamentally in their capabilities and applications. This blog post delves into the nuances of these technologies, highlighting how they unleash creativity and manage complexity in unique ways.
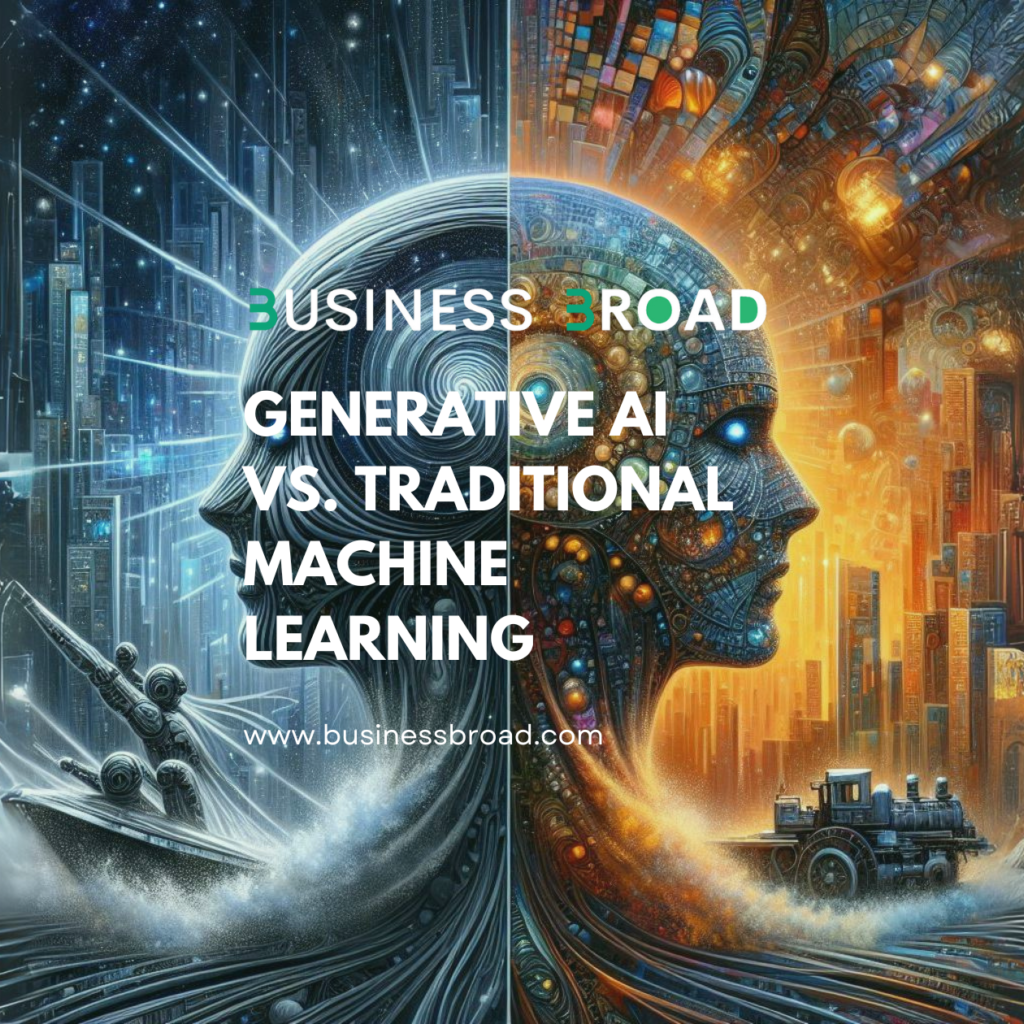
Understanding Traditional Machine Learning
Traditional Machine Learning (ML) has been the backbone of AI for decades. It encompasses a broad set of algorithms and techniques designed to make predictions or classifications based on data. These systems are adept at identifying patterns and providing insights, which makes them invaluable for applications ranging from spam detection to financial forecasting.
Characteristics of Traditional ML:
- Predictive Nature: Focuses on predicting outcomes based on historical data.
- Supervised Learning: Relies heavily on labeled datasets to train models.
- Structured Data: Excels with well-organized data in tabular formats.
The Rise of Generative AI
Generative AI represents a bold leap forward in the AI domain. Unlike its traditional counterpart, Generative AI is not just about recognizing patterns—it’s about creating new ones. This technology can generate novel data, such as images, text, or music, that mirrors the characteristics of its training set.
Key Features of Generative AI:
- Creative Output: Capable of producing entirely new content that doesn’t exist in the training data.
- Unsupervised Learning: Can learn from unlabelled data, discovering the underlying structure.
- Complex Data Handling: Thrives on unstructured data, making sense of the chaos.
Comparing Creativity and Complexity Management
When it comes to creativity, Generative AI is in a league of its own. It has the unique ability to synthesize new concepts, pushing the boundaries of innovation. This makes it particularly suited for fields like content creation, design, and entertainment.
On the other hand, Traditional ML is the go-to choice for managing complexity. Its predictive algorithms are powerful tools for making sense of intricate systems, from weather patterns to market dynamics.
Applications and Implications of Generative AI
The applications of Generative AI are vast and varied. In the realm of graphic design, it can create stunning visuals that captivate audiences. In drug discovery, it accelerates the identification of new compounds, potentially revolutionizing healthcare.
Traditional ML, with its robust predictive capabilities, remains indispensable in areas like fraud detection and risk assessment, where accuracy and reliability are paramount.
Conclusion
The debate between Generative AI and Traditional Machine Learning is not about which is better, but rather about how each can be leveraged for their unique strengths. As we continue to explore the frontiers of AI, the synergy between these two approaches will undoubtedly lead to breakthroughs that were once the realm of science fiction.
This exploration of Generative AI and Traditional Machine Learning showcases the remarkable potential of AI to drive progress. By understanding their differences and complementary nature, we can better harness their power to unlock new levels of creativity and manage complexity with unprecedented finesse.
Roadmap to Learn AI in 2024: A Complete Expert Guide
Generative AI is coming for healthcare, and not everyone’s thrilled
4 Comments
[…] Generative AI vs. Traditional Machine Learning […]
[…] Generative AI vs. Traditional Machine Learning […]
[…] Generative AI vs. Traditional Machine Learning […]
[…] Generative AI vs. Traditional Machine Learning […]